Everything is obvious—once you know the answer.
Or so I thought.
I felt a tad disingenuous when I wrote PSA: ChatGPT is the trailer, not the movie because I had already seen the movie. Due to our affiliation with Casetext and our work with the likes of Darth Vaughn at Ford Motor Company, we not only had a sneak preview of (what we did not know at the time was) GPT-4 but a front-row seat to the real-world experiments that became CoCounsel and ensured GPT-4 was ready for primetime. We, however, could not share this until GPT-4 was publicly released. We’ve been waiting, impatiently.
Serendipity smiled upon us in terms of timing. OpenAI elected to make their GPT-4 announcement at same time as an already scheduled webinar Real Talk Beyond ChatGPT: What Recent AI Advances Mean for Legal (recording, coverage) that included Darth, me, and Casetext co-founder Pablo Arredondo. Pablo was able to break the news live to a record-setting audience. GPT-4 hadn’t just arrived, it had already passed the bar exam with flying colors and had been expertly tailored by Casetext to all manner of legal use cases through extensive field testing and iteration with the likes of Darth/Ford.
This should have been joyful occasion. Instead, I was full of dread. Intellectually, I recognized we were in for a torrential downpour of inanity. But, emotionally, I was still tethered to the vain hope that once people knew what I knew, they would think like I think.
I set myself up to be disappointed despite my awareness of what was coming. I had concluded my PSA piece thusly:
I am a cautious optimist. I’ve never been more excited about what’s possible. I’ve never been more pre-annoyed at how much nonsense is about to be unleashed. It will be fun. It will also be not fun.
Unfortunately, I was not wrong.
Good-faith disagreement and rigorous analysis remain welcome. While I am quite bullish on this next generation of AI, skepticism is intellectually defensible. Indeed, interrogating the efficacy of specific applications of specific models to specific use cases is intellectually essential given the near certainty of an avalanche of poorly crafted products premised on AI magic. All of us should operate from some level of baseline skepticism.
Due to the long history of AI hype cycles, there is earned wisdom in being unaffected by the breathless coverage of new shiny objects. Even beyond potential dangers, an engaged skeptic can point to analyses from the likes of Gary Marcus and Yann LeCun on how the current approaches to deep learning have not overcome fundamental problems that have plagued AI research. I have no issue with anyone who starts from “I’ll believe it when I see it” with it being relevant, empirically validated use cases.
We would do well to heed the advice of Legaltech Hub’s Nicola Shaver’s in How to Evaluate Large Language Models for Safe Use in Law Firms:
Something happens with any technology that is subject to significant hype in the market: instead of carefully selecting a solution to solve an expressed need or to address a specific use case, organizations clamor to use the technology in any form with no specific use case in mind because the hype creates a kind of imaginary pressure to do so. It happened with blockchain, with “AI” when it first arrived in legal in the form of machine learning and natural language processing, and it happened with no-code solutions. We are now seeing the same thirst to use the current batch of advanced LLMs in law firms.
In order to get real value for a solution that is underpinned by a large language model, it is generally better to proceed by first having an understanding of the use case for which you want to use the technology, the severity and urgency of the pain-point, and then selecting the solution accordingly. There’s no point in investing in technology unless it solves a problem or adds value for your users.In order to get real value for a solution that is underpinned by a large language model, it is generally better to proceed by first having an understanding of the use case for which you want to use the technology, the severity and urgency of the pain-point, and then selecting the solution accordingly. There’s no point in investing in technology unless it solves a problem or adds value for your users.
To determine whether a specific application adds sufficient value, we also need baselining exercises like How is GPT-4 at Contract Analysis? from Zuva’s Noah Waisberg, who digs into not only how well GPT-4 performs out of the box against current contract analysis tech but also highlights the critical issue of price point (GPT-4 is relatively expensive and subject to rate limits).
Moreover, even beyond the necessary efficacy-cost analysis, there are completely valid reasons to doubt LLMs will have immediate, seismic impact.
Bets are a tax on bullshit. As discussed in my PSA, I have a friendly wager with Alex Hamilton. Alex publicly bet me that AI will notdisplace 5% of what lawyers currently do in the contracting process within 5 years. I took the other side and, frankly, would have done so had Alex proposed 30% within 3 years.
But I was trading on insider information. When I was finally permitted to disclose my advance access, I offered Alex the opportunity to cancel our bet. He declined:

Quite reasonably, Alex is banking on Martec’s Law: “technology changes exponentially, but organizations change logarithmically.”
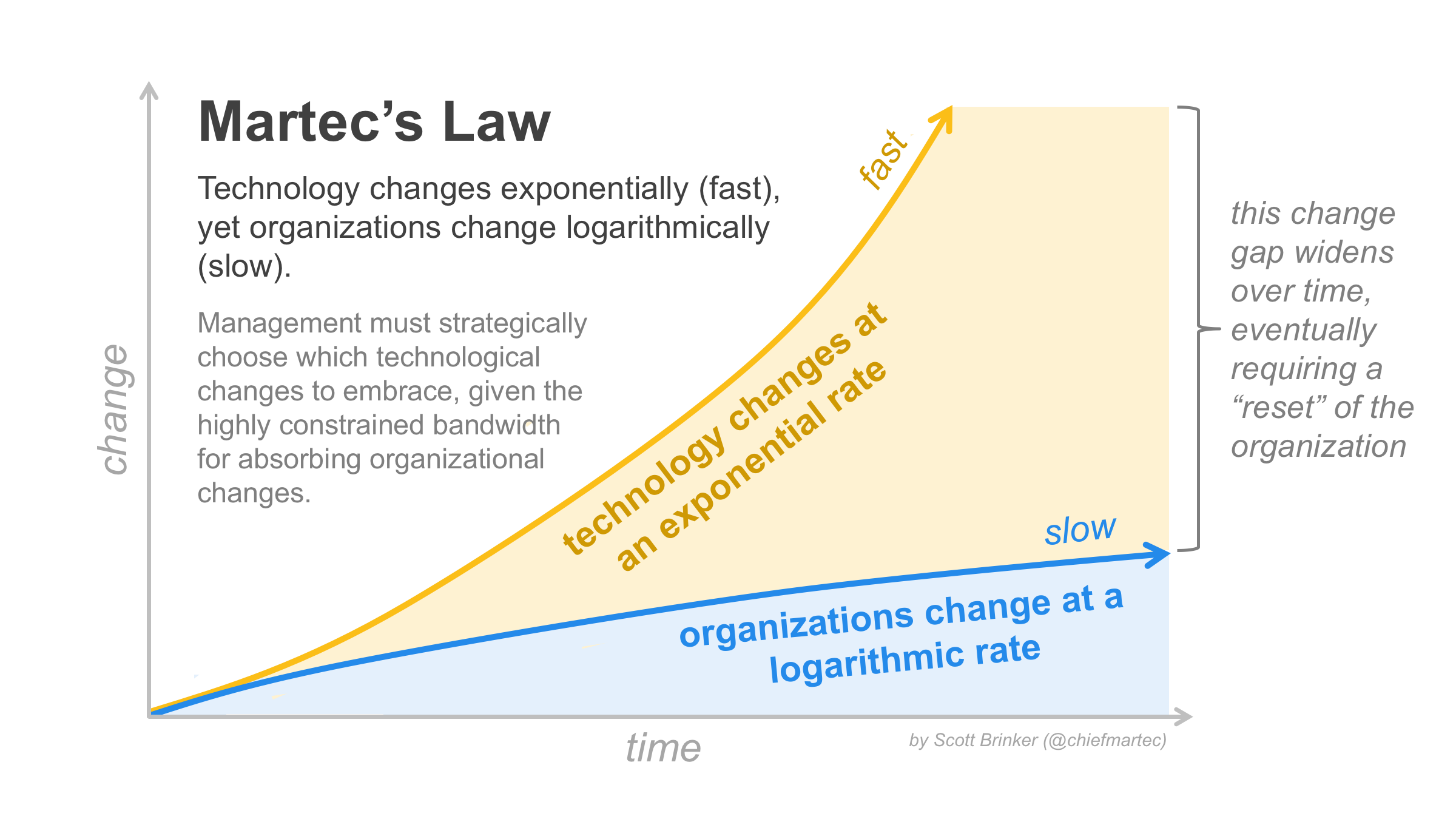
Specific to the adoption of AI, a stellar 2017 paper Artificial Intelligence and the Modern Productivity Paradox: A Clash of Expectations and Statistics investigates why advances in technology are not reflected in the productivity statistics. Of four potential causes—false hopes, mismeasurement, redistribution, and implementation lags—the authors conclude that implementation lags are the largest contributor to the paradox:
There are two main sources of the delay between recognition of a new technology’s potential and its measureable effects. One is that it takes time to build the stock of the new technology to a size sufficient enough to have an aggregate effect. The other is that complementary investments are necessary to obtain the full benefit of the new technology, and it takes time to discover and develop these complements are and to implement them. While the fundamental importance of the core invention and its potential for society might be clearly recognizable at the outset, the myriad necessary co-inventions, obstacles and adjustments needed along the way await discovery over time, and the required path may be lengthy and arduous. Never mistake a clear view for a short distance.
Those complementary investments are not merely acquisition of adjacent tech, they are investments in “human capital” and “organizational changes”—i.e., different people working in different ways. In theory, different people can be different because of re-training. In practice, different people are frequently different people, in the literal sense, because individual resistance to change borders on the suicidal (when doctors tell heart patients they will die if they don’t change their habits, only one in seven can follow through).
To be slightly less pedantic, there are quite comprehensible reasons why so many hotel rooms still feature clocks with 30-pin chargers despite Apple having sunset that tech a decade ago.
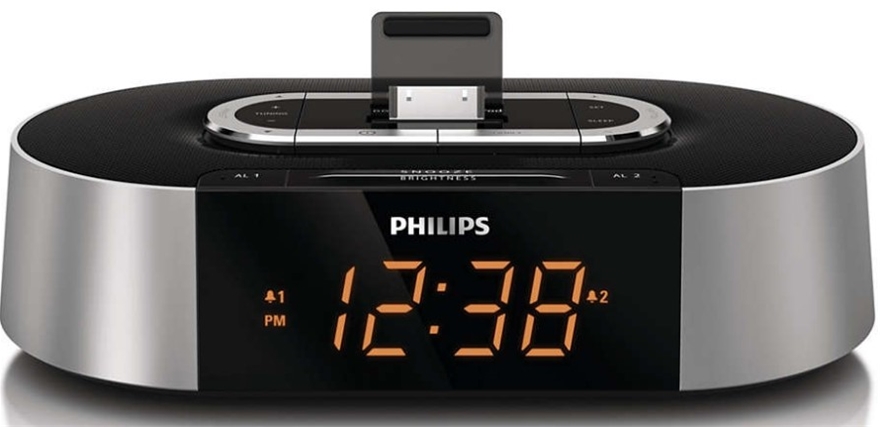
I know this. I’ve written extensively about barriers to adoption (e.g., here, here). Yet I’m betting we are entering a period of punctuated equilibrium. Punctuational change, however, is rare. I could turn out to be quite wrong. In which case, I will happily buy Alex a drink:
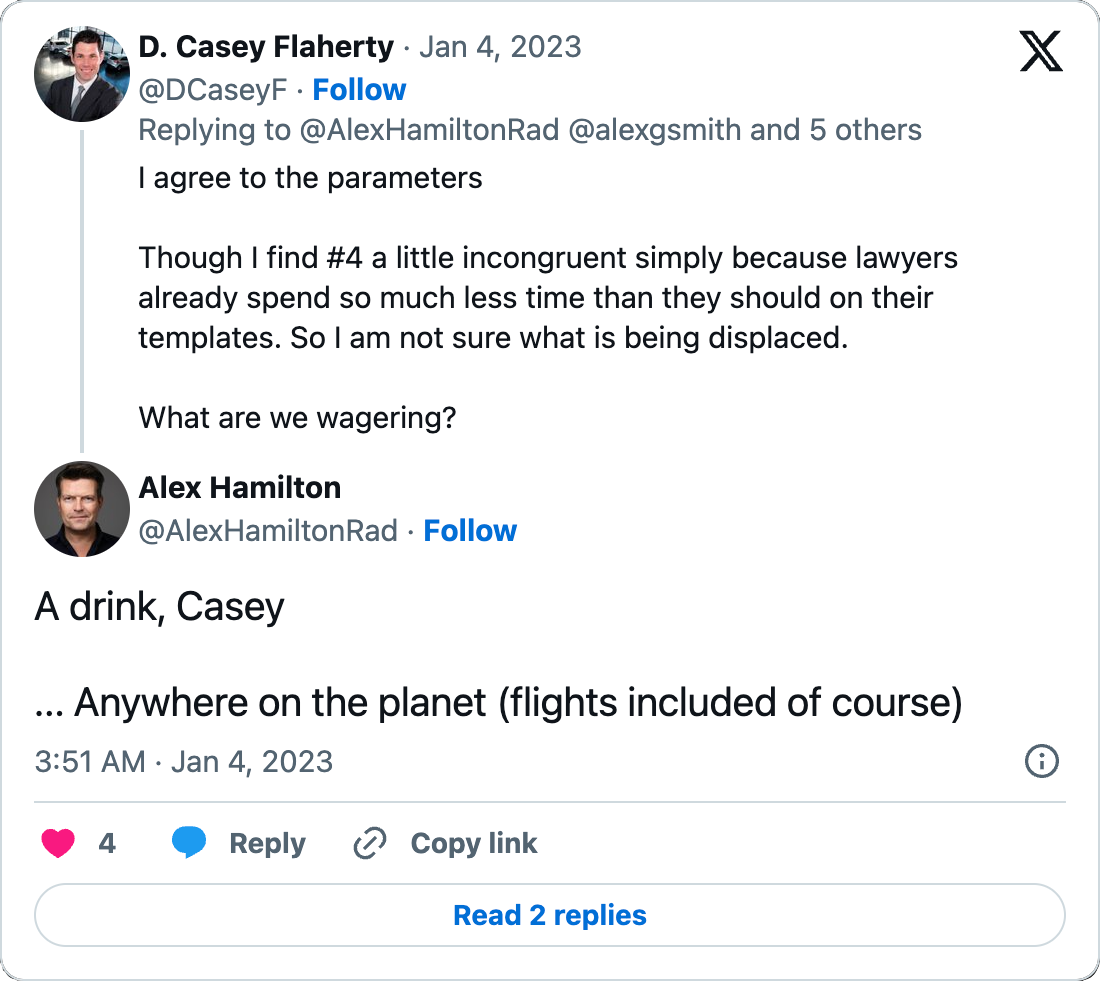
I was so taken with the wonderful stakes Alex proposed that Mike Haven (Head of Legal Operations at Intel, President of CLOC) and I adjusted our wager on a different topic to similar terms (on va boire ensemble à Montréal). These bets reflect the wonderful reality that I have all manner of minor differences with people I greatly admire. In fact, I first met Alex by disagreeing with him on the Legal OnRamp message boards 15 years ago. We’ve been debating, and friends, ever since. Betting on an uncertain future is part of the fun, and the danger, of playing close to the edge of innovation.
In brief:
- Skepticism re LLMs is warranted and necessary
- My bullishness re LLMs might be wrong (but I am betting I am right)
- Disagreements re LLMs are not to be taken personally
With that, we return to the always productive exercise of arguing with people on the internet.
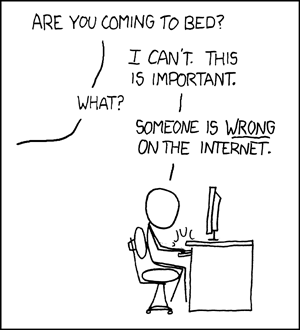
We will all just need to learn to live with the knowledge that you are not impressed. I was tickled at how news of AI passing the bar exam has allowed people to hold forth on the oddity that is American legal education.
To be admitted in most US jurisdictions, one must (i) attend multiple years of post-graduate schooling and (ii) pass a grueling exam. But the schooling does not prepare you for the exam—if you can afford it, you embark on a second cram course of study between graduating and sitting for the exam. And neither the schooling nor the exam prepare you to practice law. Oh, and the continuing legal education system is also badly broken.
Still, the bar exam—like the LSAT (which GPT-4 also did quite well on)—is a familiar and digestible benchmark. As Professor Dan Katz told Legaltech News, “I don’t even really care about the bar exam, per se. This crystallizes what is happening for people in a way [that says], here’s some tasks that lawyers do, and [GPT-4] does it marginally better.” Dan and Pablo (Casetext cofounder) teamed up with Mike Bommarito (Dan’s partner in 273 Ventures) and Casetext’s Shang Gao to conduct the bar-exam study and author the attendant paper:
In this paper, we experimentally evaluate the zero-shot performance of a preliminary version of GPT-4 against prior generations of GPT on the entire Uniform Bar Examination (UBE), including not only the multiple-choice Multistate Bar Examination (MBE), but also the open-ended Multistate Essay Exam (MEE) and Multistate Performance Test (MPT) components.
Without any domain-specific data or tuning, GPT-4 scored in the top 10th percentile, including on the written components. By contrast, GPT 3.5, the model underpinning ChatGPT, was previously tested only on the multiple-choice component and scored in the bottom 10th percentile. The latter was considered impressive at the time—the time, which now seems ages ago, was January 2023.
The world is speeding up. We need to start acclimating to the profound implications of non-linear progress. To update an example from my PSA, the AI art-generator Midjourney released its new version on March 15, the day after GPT-4 dropped. Using the prompt, “lawyers worried about robots taking their job” below is the model’s progression in a single year from version 1 (March 2022) to version 5 (March 2023):

The progress is astounding (to me, at least).
Similarly, six months ago, the idea of a machine passing the written portion of the bar exam, let alone without being specifically designed to do so, seemed like science fiction because legal language is complex, nuanced, and uncommon. Historically, “computational technologies have struggled not only with natural language processing (NLP) tasks generally, but, in particular, with complex or domain-specific tasks like those in law” due to the “the nuances of legal languages and the difficulties of complex legal reasoning tasks.”
Yet here we are. Some people, however, are not the least bit impressed.
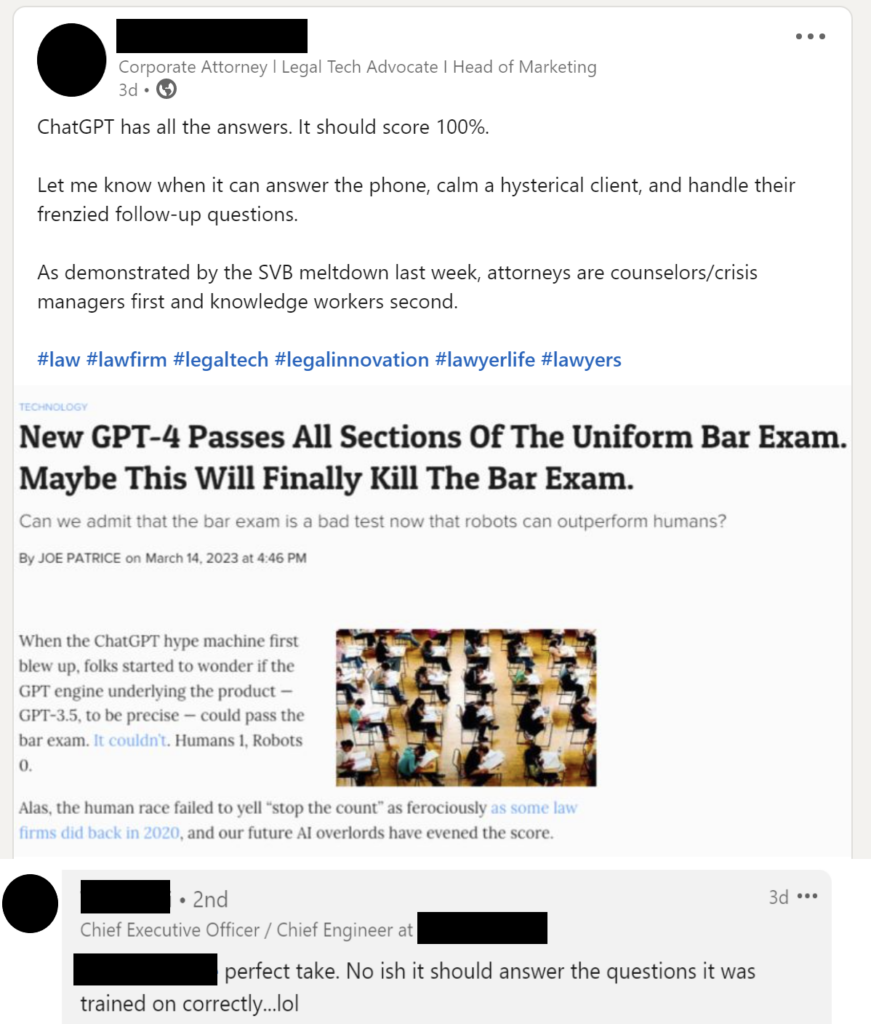
I’ve blacked out names because I have no interest in shaming anyone. But I also dislike arguing with strawmen. I am grateful for such a concrete example of bad takes I’ve seen sprayed all over the internet.
First, the reaction is factually inaccurate. This was zero-shot performance. The model was not fed domain-specific data nor tuned for the purpose of passing the exam. Moreover, the notion that LLMs have “all the answers” seems to be a common misconception. LLMs like GPT-4 do not retain access to their underlying training data, let alone have live access to the internet. This is why raw LLMs hallucinate, and why many applications will need to pair LLMs with sources of truth (i.e., non-parametric knowledge bases like the common law). GPT-4 sat for the bar exam “closed book” just like the rest of us. Still, I concede that LLMs have now demonstrated marginal advantages over humans on the tasks tested—that is the whole point.
Second, this take is surprising because the poster and commenter work at the same legaltech company. They should certainly know better with respect to the tech (the commenter is both CEO & Chief Engineer).
Until I reflected on the sense of insecurity it must trigger, I was shocked a legaltech company would greet the news of technological breakthrough that can pass the bar exam out of the box with “let me know when it can answer the phone.” I doubt the company turns up at pitch meetings and suggests their own tech is not worth exploring because “attorneys are counselors/crisis managers first.” The closest they likely come is explaining that their tech will accelerate the completion of specific tasks attorneys currently perform and thereby free up attorney time to devote to counseling and crisis management. This is a valid point. But it only underscores that advances in the automation and augmentation of specific tasks that currently consume finite attorney bandwidth merit our attention, as opposed to a “let me know when” dismissal.
Third, in this vein, the bar exam supposedly tests minimum competence—that is, the “knowledge and skills that every lawyer should have before becoming licensed to practice law,” including “a candidate’s ability to think like a lawyer.” The capacity to serve as a counselor to a hysterical client is nowhere among the admission requirements. Maybe it should be. But having reviewed hundreds of millions of dollars in legal bills, I can’t say I recall ever having seen “calming a hysterical client” among the narratives.
While the counseling aspect of lawyering is critical, it is not where the majority of lawyers spend a majority of their time (limiting my comments to BigLaw and in-house). Neither the article nor the underlying paper come anywhere close to the ultra-strawman of suggesting GPT-4 immediately replace all lawyers. The impact of these advances should be framed in terms of tasks, not jobs, with measurements calibrated to return on improved performance, not headcount reduction.
These were patently poor takes. Yet, until I chimed in, the comments to the post were universally positive. Even in putatively sophisticated legaltech circles, confirmation bias and echo chambers are in full effect in ways that are stunting the discourse. My (least) favorite example:
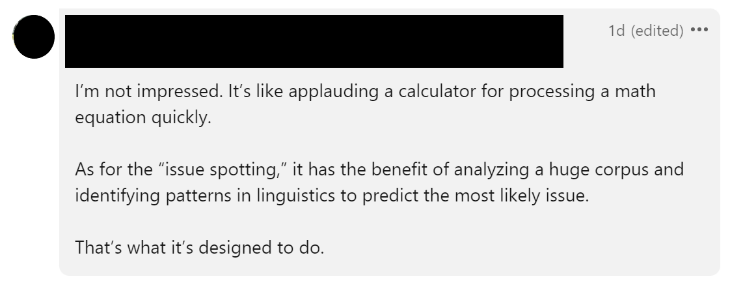
While we just have to live with the disappointment of not impressing this commentator, the historical record suggests that Blaise Pascal’s calculator did impress a few people in 1642—and for centuries thereafter. Indeed, it was considered such a monumental achievement with “such a profound influence upon applied mathematics and physics” that the tercententary celebration was held in London because the inventor’s native France was then under German occupation. But, no doubt, some hard-to-impress people responded to the calculator with a yawn and proclamation analogous to ‘let me know when it can pass the bar exam.’
Stay Informed, Stay Ahead
Discover the latest trends and insights in the legal industry. Learn more about Generative AI, Delivering Legal Services and Strategy and Scale in a Complex World.
Let’s Forge the Future Together
Interested in joining our roster of legal innovators or simply curious about the world of legal tech? Reach out! Our team is eager to connect, collaborate, and contribute to the ever-evolving legal landscape.
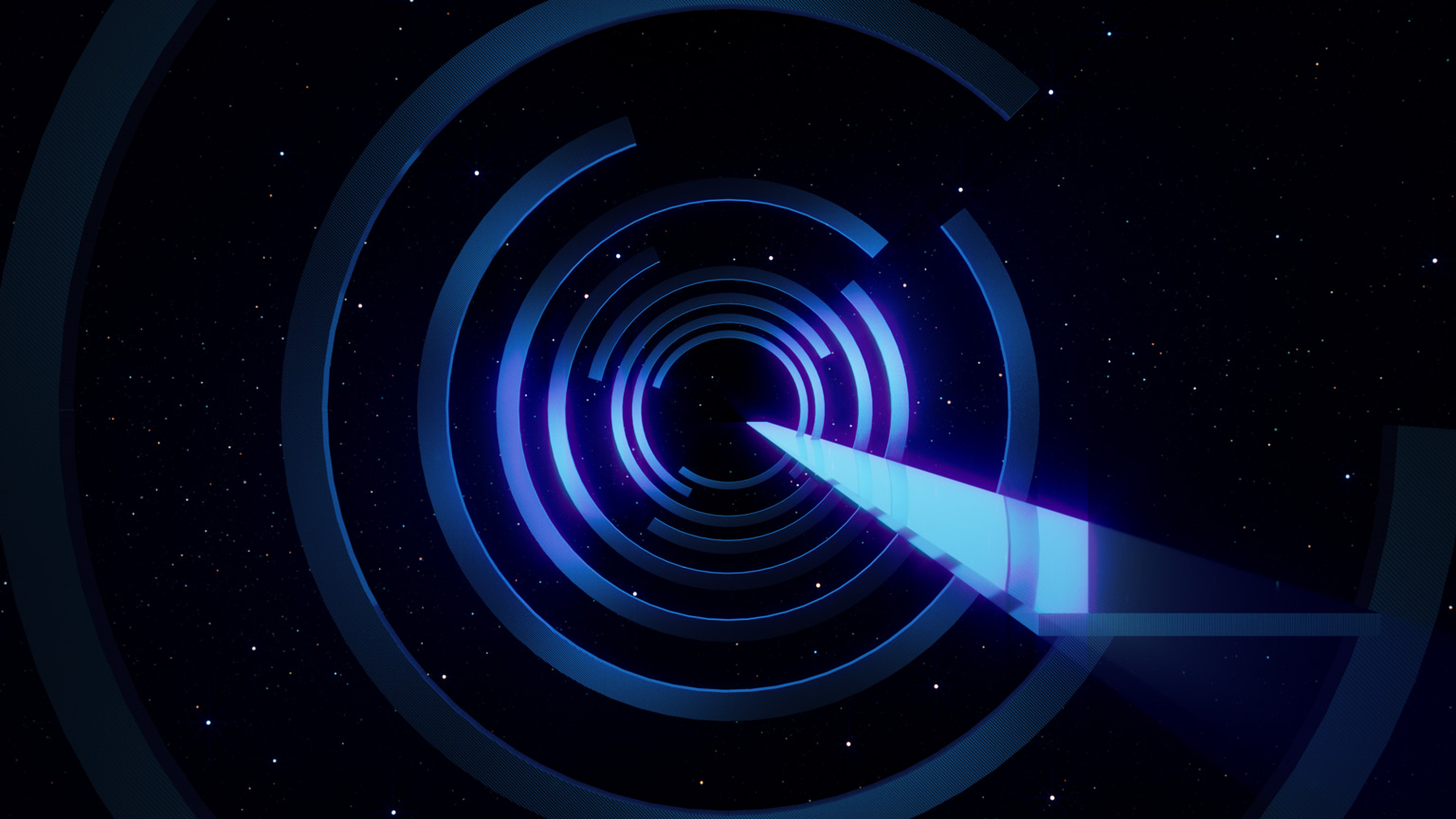